The key to cutting-edge target marketing lies with the integration of two data mining initiatives:
- The determination of whom to promote, via statistics-based predictive models. This is a binary ""yes/no" "process involving the estimation of future purchase activity.
- Insight into what to promote, using four quantitative techniques: tree analysis, demographic profiling, focus groups, and survey research.
Few catalogers have attained this sophisticated level of target marketing. Instead, they are mired in the traditional world of RFM Cells.
This article will provide a framework for integrating multiple analytical tools into a sophisticated program of differential catalog marketing. It will focus on a mid-sized cataloger with merchandise geared mostly to women; actually, a composite of several current and past clients.
RFM Cells "Original Customer Classification System
According to Catalog Age's 1998 Benchmark Report on Lists and Databases, over one-half of catalogers still rely on RFM Cells to make promotional decisions. Until recently, the same was true of our mid-sized cataloger. Accordingly, just three characteristics were used to classify customers:
- Recency , or the number of months since the most recent paid order. Each customer was placed in one of four possible monthly categories: 0-6, 7-12, 13-24 or 25+ months since the last paid order.
- Frequency , or the total number of historical orders. Customers were classified by 1, 2 or 3+ previous paid orders.
- Monetary , or historical Average Order Size. Our cataloger created three equally-sized groups ""Low," "Mid," and "High."
The result was 36 (4 x 3 x 3) Recency/Frequency/Monetary permutations, or RFM Cells.
RFM Cells "Determining Whom to Promote
Let's examine how our cataloger used these 36 RFM Cells to determine whom to promote. First, some assumptions:
- The cataloger is willing to mail to breakeven, and no further. Breakeven was "and is "$1.25 per piece mailed.
- Generally, a re-mail is done several weeks after the initial drop, with a response rate that is about one-half of the first.
To determine which customers to promote, the cataloger examined historical results in order to develop by-Cell estimates of future purchase volume. Then, it created a hierarchy of cells, from highest to lowest estimated performance. Those cells that were expected to do at least $1.25 per- piece-mailed were contacted. Likewise, those at $2.50 or more on the initial drop were mailed a second time.
RFM Cells "A Crude Technique for Selection
Consider the Frequency input to our cataloger's 36-Cell segmentation strategy. Customers who have purchased 10 times, for example, have been categorized identically to those who purchased three times. In reality, they have been much more loyal and should have been designated as such. However, significantly increasing the number of categories to accommodate these differences reduces each Cell's sample size to such an extent that it becomes difficult to determine the RFM hierarchy.
This problem is compounded exponentially if we include into the RFM Cells other key database fields such as:
- Merchandise categories.
- Satisfaction indicators such as returns, exchanges and allowances.
- Service measures such as backorders and out-of-stocks.
- Payment indicators such as cash versus credit, and phone versus mail.
- Overlay demographics such as age, income, marital status and presence of children.
Clearly, an RFM strategy that took full advantage of our cataloger's comprehensive customer database would require thousands "and perhaps tens of thousands "of Cells. This would be an unwieldy strategy to implement, and one with many opportunities for error. And, Cell sizes would be far too small to create a stable RFM hierarchy.
Statistics-Based Predictive Models "A Selection Tool for Today's Sophisticated Databases
Statistics-based predictive models do not have these limitations. All database fields with the potential to separate future buyers from non-buyers can be input to the modeling process. There are none of the sample-size issues that are inherent in RFM Cells. And, the result of the model "a rank-ordering of customers by predicted future purchase volume "results in a straightforward implementation. All customers above a predetermined predicted performance are promoted, and the balance are not.
In short, predictive models are more stable than RFM Cells. They're easier to implement. And, they do a substantially better job of determining future purchase behavior.
The Superiority of Statistics-Based Predictive Models "An Example
Table 1 shows the performance of a predictive model that replaced our mid-sized cataloger's traditional RFM segmentation strategy. Recall that our cataloger promotes to $1.25 per piece mailed, and experiences a 50% fall off with re-mails. All customers have been:
- Scored by the model.
- Rank-ordered from highest to lowest predicted performance.
- Grouped into ten equal performance segments ("Deciles").
Table 1: Customer Predictive Model
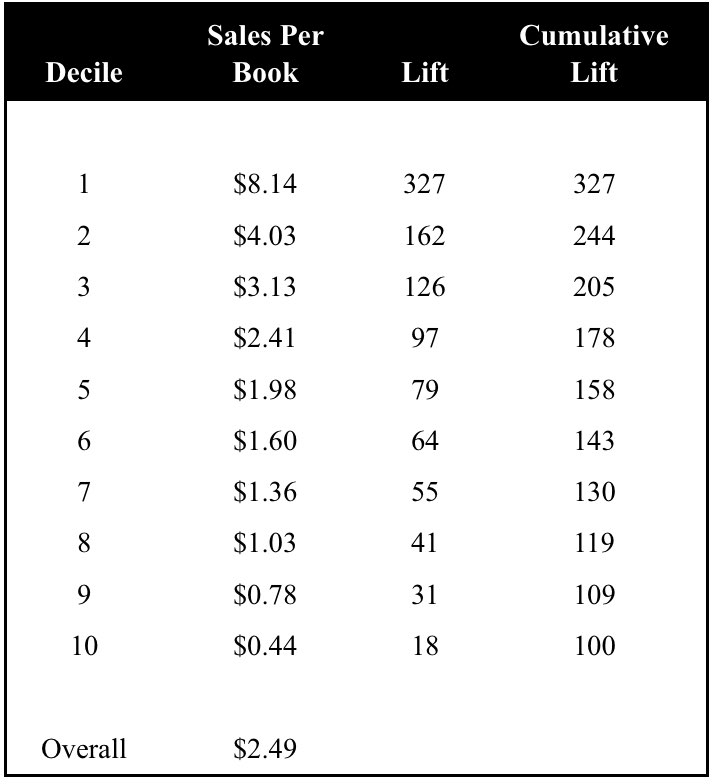
Customers in Decile 1 generate $8.14 per piece mailed, while Decile 10 brings in just $0.44. Combined, all of the Deciles average $2.49. The Lift column shows the performance for each Decile compared with this $2.49 average, while the last column records the cumulative ("running") lift. For example, Deciles 1 through 5 have a cumulative lift of 158. This means that, if the cataloger limited a promotion to just this group, the results would be 58% better than the $2.49 average for the entire file.
Table 2 illustrates the incremental power of the predictive model versus the traditional RFM Cells:
Table 2: Customer Predictive Model
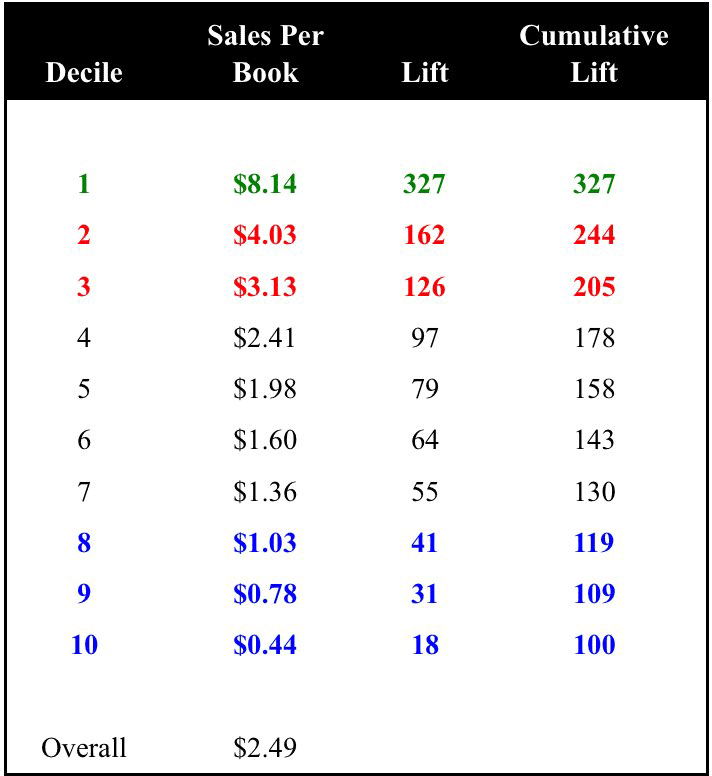
- Deciles 8 through 10 all are below our $1.25 breakeven, and should be eliminated from future promotions. Our cataloger's original RFM Cells, on the other hand, were only able to identify about 10% of the customer base as being unprofitable. With the statistics-based predictive model, the money that is freed up by eliminating additional unprofitable circulation can be allocated to profitable re-mails, as well as to innovative targeted marketing programs (more on this shortly).
- Deciles 1 through 3 can be re-mailed. Even with a 50% performance drop-off, dollars per piece mailed will still be over $1.25. RFM Cells were only able to identify about 15% of the database as being re-mailable.
- Decile 1 performs so well that it can be re-mailed twice. That's because the resulting performance "even after a 75% drop-off "remains comfortably above the $1.25 breakeven. The RFM Cells were unable to identify any customers who profitably could be re-mailed twice.
Additional Analytical Tools "Determining What to Promote
We've seen how statistics-based predictive models are superior to RFM Cells for determining whom to promote. However, there's much more to state-of-the-art database marketing than optimally identifying the mailable portion of customers. We also need to determine what to promote, via customized catalogs. And, to do so requires the use of additional analytical tools. This is because statistics-based predictive models, for all of their power, create what is known as heterogeneous segments; that is, segments containing individuals with multiple characteristics.
Decile 1 of our mid-size cataloger's predictive model, for example, contains men and women, as well as the old and the young. In addition, it includes customers who have purchased many different combinations of merchandise. In fact, these customers have just one guaranteed similarity: they are expected to order an impressive amount of merchandise in the future.
Tree Analysis "Identifying Multiple Types of Customers
Tree analysis, on the other hand, creates homogeneous segments; that is, each segment contains individuals with identical merchandise-purchase and/or demographic characteristics. The following are two hypothetical tree analysis segments:
- 40-50 year old female jewelry buyers, with four or more purchases, averaging $500+, and at least one purchase within the past six months.
- 30-35 year old male electronics buyers, with three or more purchases, and at least one within the past twelve months.
The beauty of tree segments is that they provide us with marketing insight. Feed these descriptions to a good creative staff and the result will be a brainstorming session on how to tailor the catalog to the demographic characteristics and product interests of multiple customer groups.
There are many ways to tailor a catalog. Perhaps the simplest are ink-jet messaging and "blow-ins." More involved techniques are over-wraps, customized covers, and "glue-ins." The ones that require the most up-front investment are selective binding and specialty books.
Tree Analysis "An Example
In order to identify multiple types of customers, our cataloger ran a tree analysis off database fields limited to merchandise categories and demographics. These are the most likely to offer clues on how to match the content of a catalog to a given customer's needs. Other variables "recency, frequency, average order size, and the like "are helpful for predicting future purchase behavior. However, they provide little insight into customer lifestyles or interests.
The results our cataloger's tree analysis are illustrated in Table 3. We see that female customers generate sales-per-piece-mailed of $2.88, over twice that of the males' $1.42. However, the analysis reveals a small pocket of male customers who, at $2.95 per piece mailed, are actually more responsive than the average female. These are buyers of jewelry merchandise.
Our cataloger's marketing staff, intrigued by this finding, set out to uncover additional clues about the purchase dynamics of this profitable customer segment. Accordingly, an analysis was performed to determine the types of jewelry merchandise being purchased. In almost all cases, it was merchandise targeted for females. Two plausible hypotheses were developed:
- The actual purchasers are women who are using credit cards that are in the names of their husbands.
- They are men purchasing gifts for the significant women in their lives.
Profiling "Developing Portraits of Your Customers
Our cataloger's next investigative step was to send its customer database to an overlay company for a demographic and psychographic enhancement. As a result, scores of fields were added to each customer's record, including age, income, marital status, and presence of children.
The results suggested that these jewelry buying households "regardless of whether the purchase is being made by a card-toting wife or a gift-giving husband "are leading "Ozzie & Harriet" lifestyles. These are families with children, living in single family suburban homes, with professional, technical and managerial occupations.
Knowing that these are married suburbanites rather than single city-dwellers will be helpful in tailoring future catalog copy. Nevertheless, our cataloger still didn't know if the purchaser is the husband or the wife. To gain a definitive answer, it commissioned a series of focus groups as well as some comprehensive survey research.
Focus Group and Survey Research "Developing Psychological Insight into Jewelry Customers
The focus group and survey research determined that the majority of these individuals are gift-giving husbands. They are what the research company dubbed "unimaginative male gift givers." These are men who "despite their solid professional success "dread buying birthday, anniversary and holiday gifts for their spouses. They very simply are at a loss for what kinds of presents their wives might find appealing.
In order to fully leverage these findings, our cataloger convened a task force. Comprised of representatives from Marketing, Creative, and Analytical, the task force's mandate was to develop a loyalty program to appeal to these "unimaginative male gift givers." Based upon the insight gained from the research, the loyalty program was built around the following key features:
- Automatic reminders for upcoming birthdays, anniversaries, and other personal milestones. This is a registry based on self-reported information, so that our cataloger can remind its participating male customers of upcoming special events that will require a gift. Based on customer preferences, these reminders can be sent via phone or e-mail.
- A consulting service for gift selection. The cataloger staffed its in-bound call center with specially trained "gift consultants." This service was tied to the existing database, to ensure that duplicate gifts are not recommended.
- All gifts are wrapped and then mailed to either the male program participant or "if he's out of town "his wife. Likewise, a card is included, either blank or fully addressed.
On the prospecting side, the cataloger's circulation department began working with its list broker to identify new male-oriented lists to target prospecting catalogs. These catalogs include a description of the loyalty program as well as a form for signing up.
Summary
Our cataloger had for many years used traditional RFM Cells to determine whom to promote. However, it recognized that statistics-based predictive models do a substantially better job of determining future purchase behavior "as well as being much easier to implement. As a result, it was able to optimize its promotional strategy, in terms of both initial and follow up mailings.
The cataloger also realized it is critical to develop deep insight into what to promote, and to establish specialized, targeted marketing initiatives such as loyalty programs. Accordingly, it employed four quantitative techniques "tree analysis, demographic profiling, focus groups, and survey research "to identify, cultivate, and grow a small but profitable group of male customers.
Over time, our cataloger "by optimizing both whom and what to promote "was able to drive significant increases in its revenues as well as profits.