Marketing analysts rely heavily on data collected in the course of daily operations and transaction files. The quantity and variety of data are far greater than what might be available from surveys or focus groups. Yet the process of data collection and maintenance is largely beyond the researcher's control. The task then is to render data usable for analysis in a timely and affordable fashion. Usable encompasses:
- Relevance: Are the available data relevant to the analysis at hand? What files have to be tapped?
- Accuracy: Does the data reflect what happened? How error prone was the data entry process? How reliable were the programs used to maintain the files?
- Completeness: Did all relevant events get recorded? Were all the connections between events reflected in the data? For how long were history records kept? Are there missing segments of data?
- Consistency: Can the data always be interpreted the same way? Were coding schemes always the same? Did the use of fields change over time? Is the degree of accuracy the same in all segments of the file?
- Appropriateness of file organization and formats: How much does the data need to be manipulated before a selected method of analysis can be directly applied to it?
Most operational systems are designed with little regard to future analytical needs. As a result, a multitude of problems are uncovered when a company attempts to use its historical data strategically.
This article will describe the most frequent of these problems and suggest ways of identifying them as well as discuss short-term and long-term solutions.
The overriding concern of data processing is operations, not marketing decision support. This is reflected in the way the data are maintained. Here are some problems we have observed and challenges they present:
- All transactions that belong to a single customer are not stored under a single account number. This happens if deduplication is done on mailing tapes, but not on the customer database as a whole. If orders are not always connected back to their originator, RFM cell analysis, a staple of circulation planning, will be diluted. If a company enjoys a high geographic penetration rate, this problem may be severe, in which case internal deduplication will be required.
- Incomplete customer data can result from other system shortcomings as well. For example, account numbers might be changed as telemarketing representatives are reassigned, or orders might be stored under a recipient's ID rather than the giver's.
- Customer and order source codes are often contaminated with wrong or garbage data because of data entry errors. Last-minute changes in a circulation plan may leave a customer falsely marked as mailed that particular promotion. Lifetime Value analysis relies on customer source codes to segment prospects and on promotion codes to estimate circulation cost. Order source codes are vital in evaluation of alternative mailing strategies. Correction of these problems may require retrieval of volumes of old mailing tapes (if available) to match names and addresses.
- Transaction files may contain order splits: multiple records created from a single purchase which involved back ordered, canceled, returned, exchanged, multimedia or special delivery method items. Analysts would need to bring these records together to create a history of each marketing transaction.
- Data that logically belongs together might be stored in several files and formats. The older data might be archived, and the most recent transactions stored in an "unfulfilled" order file. Mailing records might also be in different formats if, over time, the company used different service bureaus and key coding schemes. Analysis cannot move ahead until a uniform format is created.
- Conversely, distinctly different types of records might be kept in the same file. Customer and prospect records might be found together and, without access to all order records, it may be hard to tell inactive customers from prospects. Order files might be used for both mail order and retail credit card transactions, potentially resulting in the misinterpretation of mail orders demand or returns. Finding a method to differentiate among various record types is important. The method might be as simple as using an existing "type" field or as complex as devising a set of rules that key on several pieces of information, such as IDs, dates and amounts.
- Life-to-date counts and dollars often exist in the system to facilitate analysis, mailing selection and list rental. However, such fields often are not maintained consistently. For instance, cancellations, returns and exchanges may be included in some cases and excluded in others. The same problem is found in order summaries, such as total order amount, which may or may not include canceled items and shipping charges. As a rule, summary fields should be avoided in favor of detail.
- The coding method of some fields may have been altered as operations evolved. Fields related to fulfillment or payment status are frequently subject to this because customer service and collection procedures change. It is impossible to use these fields without recoding.
- Similarly, SKUs might be reassigned from catalog to catalog. Special SKUs might be used for promotion or discount items. Before undertaking product affinity analysis or item demand forecasting, reference tables must be built to uniquely identify products bought and incentives that were in effect as well as to assign SKUs into appropriate analysis groups.
- Some fields, notably dates, are often used for more than one purpose depending on the content of some other field. For example, the same field may contain shipping date, return date or a cancellation date depending on status. Status fields themselves often reflect only the most recent values. In effect, information is lost that could have been used to analyze cancellations and returns as well as the effect of back orders on a company's business.
Assessing the extent of the specific problems requires information from data processing and most importantly, a proactive data analysis:
- Files should be visually inspected for duplication. Sorting customers by ZIP Code and name will make this task easier. Orders should be sorted by customer ID and date to check for duplication and splits.
- Simple ratios, such as line-items-to-order and dollars-to-orders, should be checked for consistency over time to reveal gaps or duplication in the files.
- Frequencies should be run on coded fields, overall and by season, and reconciled with operational statistics, such as number of orders by status, by payment type, number of items by product category etc.
- All available financials such as demand, shipments and returns should be broken down by period and compared with accounting records. Anything that can be cross-checked should be. These checks will reveal whether, in aggregate, the data is correct.
- To verify that nothing is seriously wrong on a more detailed level, a simple RFM cell structure and report showing performance of each cell can be created. The basic RFM relationship to performance should hold across all cells with substantial customer counts. If you have prior reports of performance by customer segments, recreate them for additional checking.
These data verification techniques and problems they help detect are summarized in the table below:
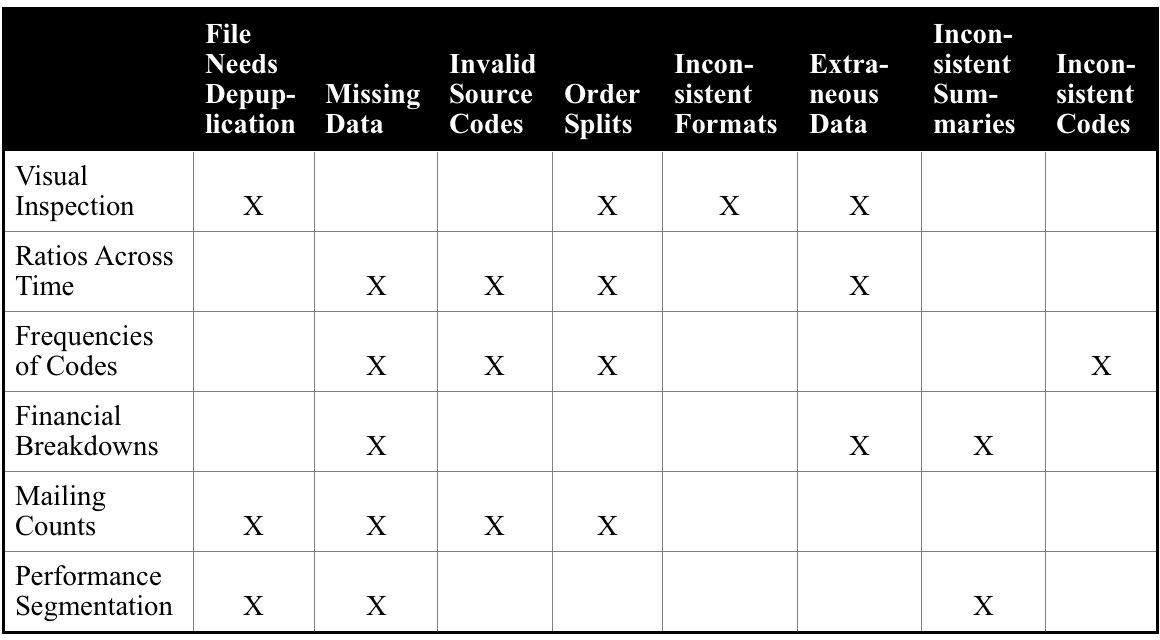
Some data problems may be too costly to fix, forcing the methods and scope of analysis to be altered. Here are areas of compromise to consider in the context of projected analysis needs:
- Consistency versus Accuracy: Any segmentation analysis involves ranking of prospects or customers based on relative performance. The accuracy of the performance measure is not nearly as important as the absence of bias to any particular segment; that is, consistency.
- Aggregate versus detail data integrity: If in segmentation your decision unit is a ZIP Code, the unbiased assignment of circulation and responses to each ZIP is sufficient. This is true even though, on a prospect level, tracking might have been poor, preventing you from doing household level modeling.
- Rules and approximations versus history of transaction detail: For example, cost of goods and other financial ratios can be used in place of individual transaction costs, if the approximation is sound. Promotional costs can be recreated by applying rules used in circulation planning.
- Analysis of major promotions-only versus all promotions: Modeling can be performed on a few major promotions that are likely to have been tracked more accurately, then applied to others.
- Access to recent periods of data versus all of a company's history: For example, product affinity analysis requires a few most-recent periods, and useful insights can be gained from just one mailing season.
- Using sample versus 100 percent of the data: Sampling can alleviate some processing headaches, without a serious impact on quality of the results, if you are not breaking data into very small segments and do not need to use the same files for mailing selects.
To avoid problems, measures can be taken in the long run to improve treatment of historical marketing data. As sophisticated, data-driven marketing becomes a necessity, one can expect management to support this endeavor. Specifically, attention should be directed toward these goals:
- Create incentives for correct data entry, particularly of source codes. The key here is careful design of the entry process and edits as well as continuous measurement of its integrity. Codes should be constructed to minimize the possibility of confusion and ease of verification. A simple comparison of one operator's entire weekly output with the expected distribution of codes will do a good job of measurement. Matching orders back to the mailing tapes would be a natural extension, although the cost may prohibit this strategy on a full scale. At a minimum, it should be done periodically on samples of incoming orders as a way of measuring reliability of the data entry.
- Ensure that all activity records related to one original transaction carry an identifier that can be used to pull them all together. In the world of relational databases this should not be hard to implement.
- Avoid discarding original transactional detail in favor of summaries. While there is a limit to how much data can be maintained online, comprehensive archiving can be done in a way that allows easy retrieval.
- Keep history of customer and transaction status changes, by archiving previous status record with its effective date range.
- Periodically deduplicate your customer database on the basis of matches found during mailing merge/purges.
- Have a mechanism for correcting promotion history records after last-minute changes in circulation plans.
- If possible, retain information commonly created during a merge/purge: ZIP correction, Carrier Route code and address correction/change of address indicator.
- Avoid multipurpose use of fields.
- In creating coding schemes, combine several codes into one only if the components represent something intrinsic and invariant. For example, catalog code, color and size could be components of an SKU because their interpretation is not likely to change. On the other hand, a product classification code should not be included (unless it designates a distinct section of the catalog), because it is subject to regular revisions. Instead, maintain reference tables that translate all codes into English and can be used to classify them.
- Ensure that source codes and offer codes are unique, even if it means embedding part of the date in it.
- Keep good computerized cross-references of changes in coding schemes.
- In addition to auditing data as part of every analytical project, arrange for it to be done periodically. Create a Data Quality Assurance function.
Even if most data integrity problems are solved at their source, the effort to bring files into formats easily manipulated in marketing analysis will be substantial. This is because the purpose, the logical view of data and the pattern of its use in an operational system differ substantially from those in a decision support environment. As more and more analysis is needed, it will pay to invest in a stand-alone comprehensive analytical database with a well-designed permanent interface to the operational system. Once built, it will allow analysts to concentrate on data analysis rather than on data preparation.
Your data is an invaluable strategic marketing resource. To derive its full value, it is critical to ensure its usability. Problems must be anticipated, data verified and methods and scope of analysis judiciously selected. The goal for the long run should be improved overall data maintenance procedures and the creation of a comprehensive analytical database.